WorldMove provides mobility datasets for over 1600 cities spanning 179 countries across 6 continents
WorldMove is an open access worldwide human mobility dataset, we follow a generative AI-based approach to create a large-scale mobility dataset for cities worldwide. Our method leverages publicly available multi-source data, including population distribution, points of interest (POIs), and synthetic commuting origin-destination flow datasets, to generate realistic city-scale mobility trajectories.
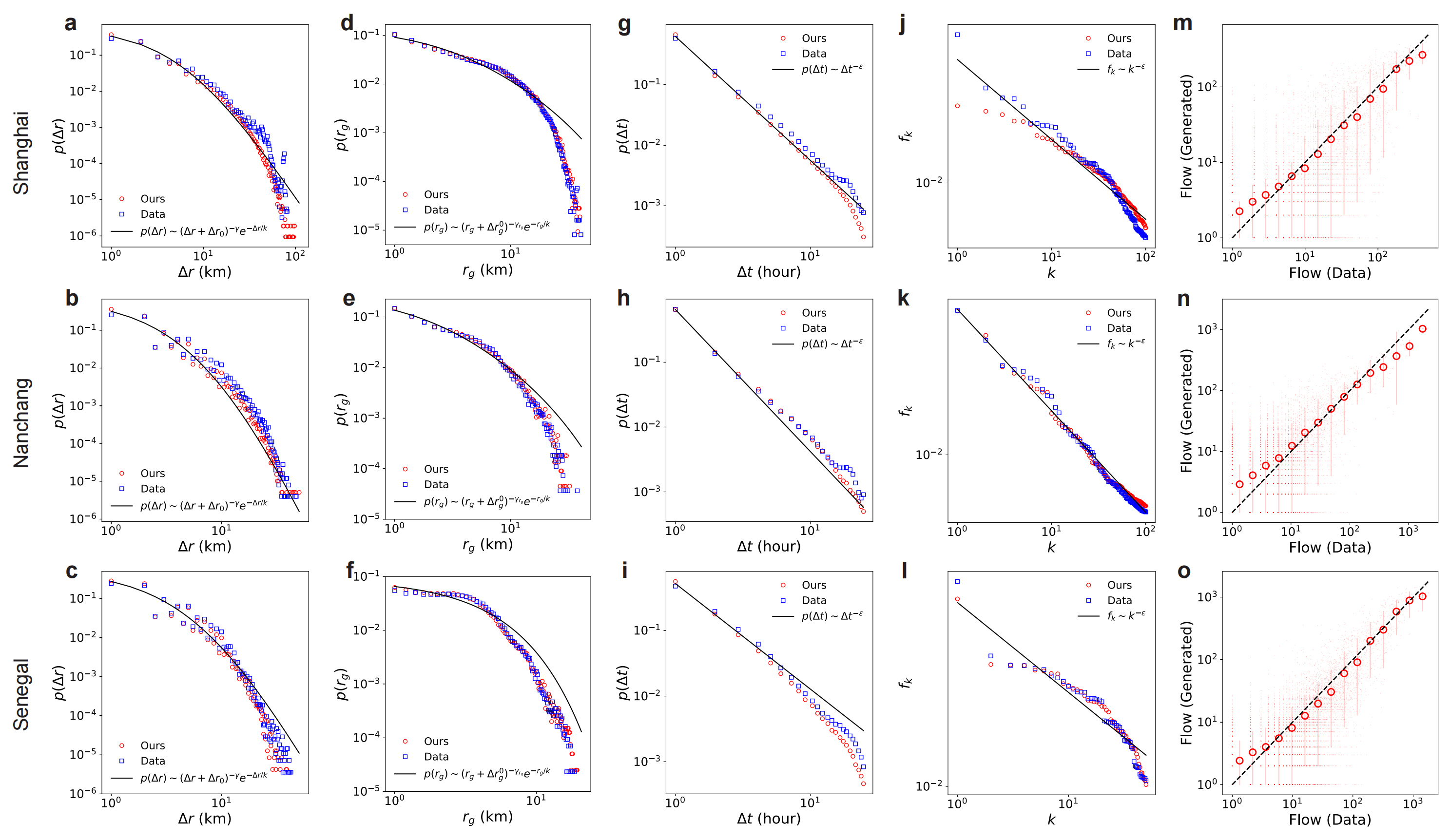
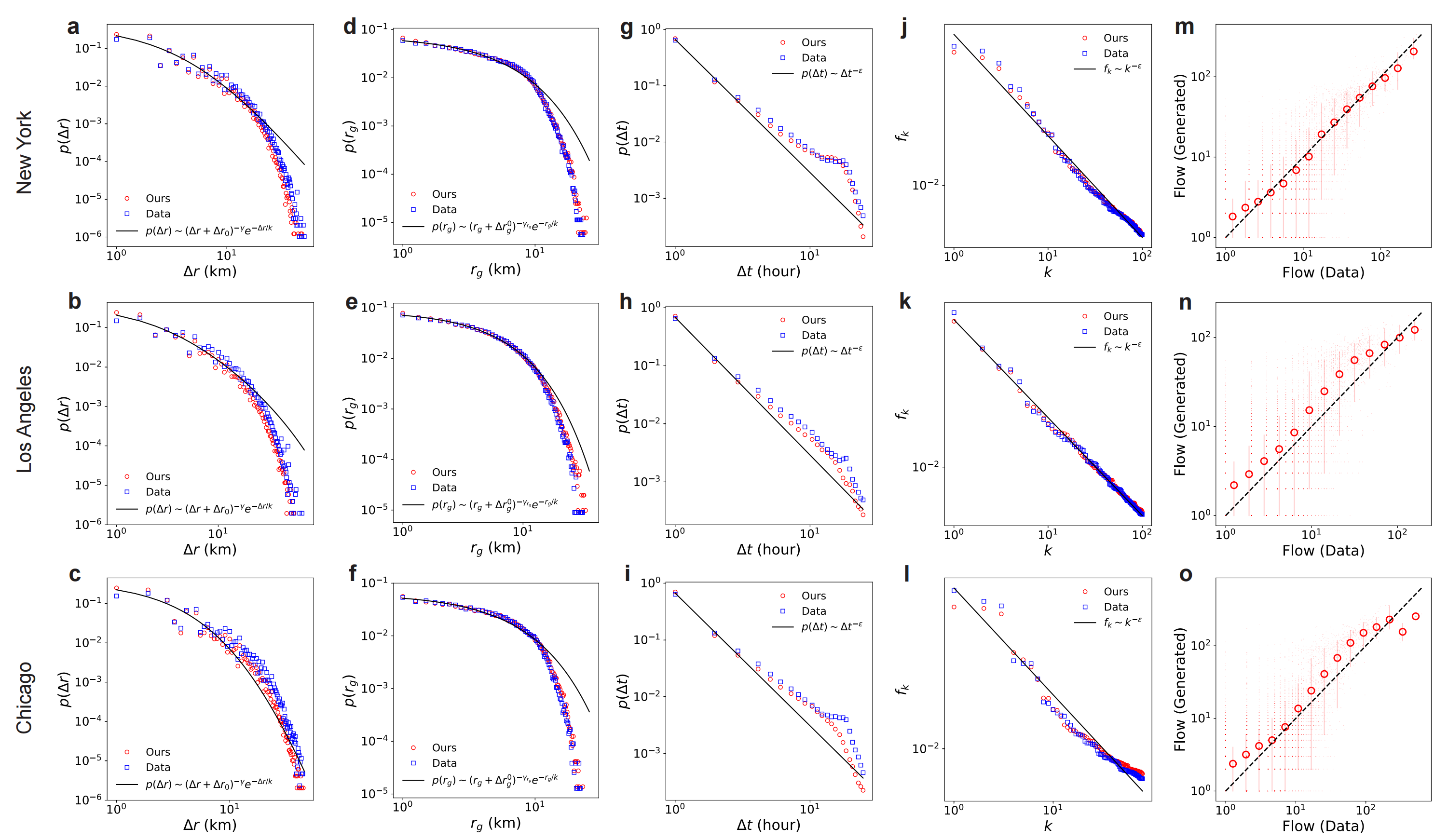
Mobility Patterns
We analyze the generated mobility patterns on 6 cities across 3 countries to evaluate whether the generated data can not only resemble real-world data but also adhere to fundamental mobility laws, including power-law of jump length, radius of gyration and wait time, Zipf's law which characterizes the frequency distribution of visited locations, and flow data which shows the collective movement patterns of human mobility.
Collective Movement
While WorldMove generates individual-level mobility trajectories, it is important to ensure that these fine-grained movements can accurately reproduce aggregated urban mobility patterns. One key proxy for such patterns is the commuting origin destination (OD) flow, which reflects where people travel from and to during typical commuting hours. To intuitively demonstrate that the generated trajectories accurately reflect commuting OD flows, we visualize the distribution of these flows during morning and evening commuting times in Figures. We include cities from various continents, including China, Africa, and the Americas, to provide a diverse range of examples.
As we can observe, the generated OD flows closely resemble the real-world patterns, indicating that the generated mobility data can capture aggregated mobility trends effectively. In addition, the generated data reflects regional heterogeneity in commuting structures. For example, in large metropolitan areas such as Shanghai and several U.S. cities, we observe clear directional flows from peripheral residential zones to central business districts in the morning, and the reverse in the evening—patterns typical of centralized urban employment. On weekends, this structured pattern becomes less prominent, consistent with reduced work-related travel. In contrast, in medium-sized cities such as Nanchang, China, where residential and workplace zones are less spatially segregated, the OD flows are more evenly distributed. The generated data accurately captures these distinctions, further validating its ability to reproduce nuanced urban commuting behaviors.
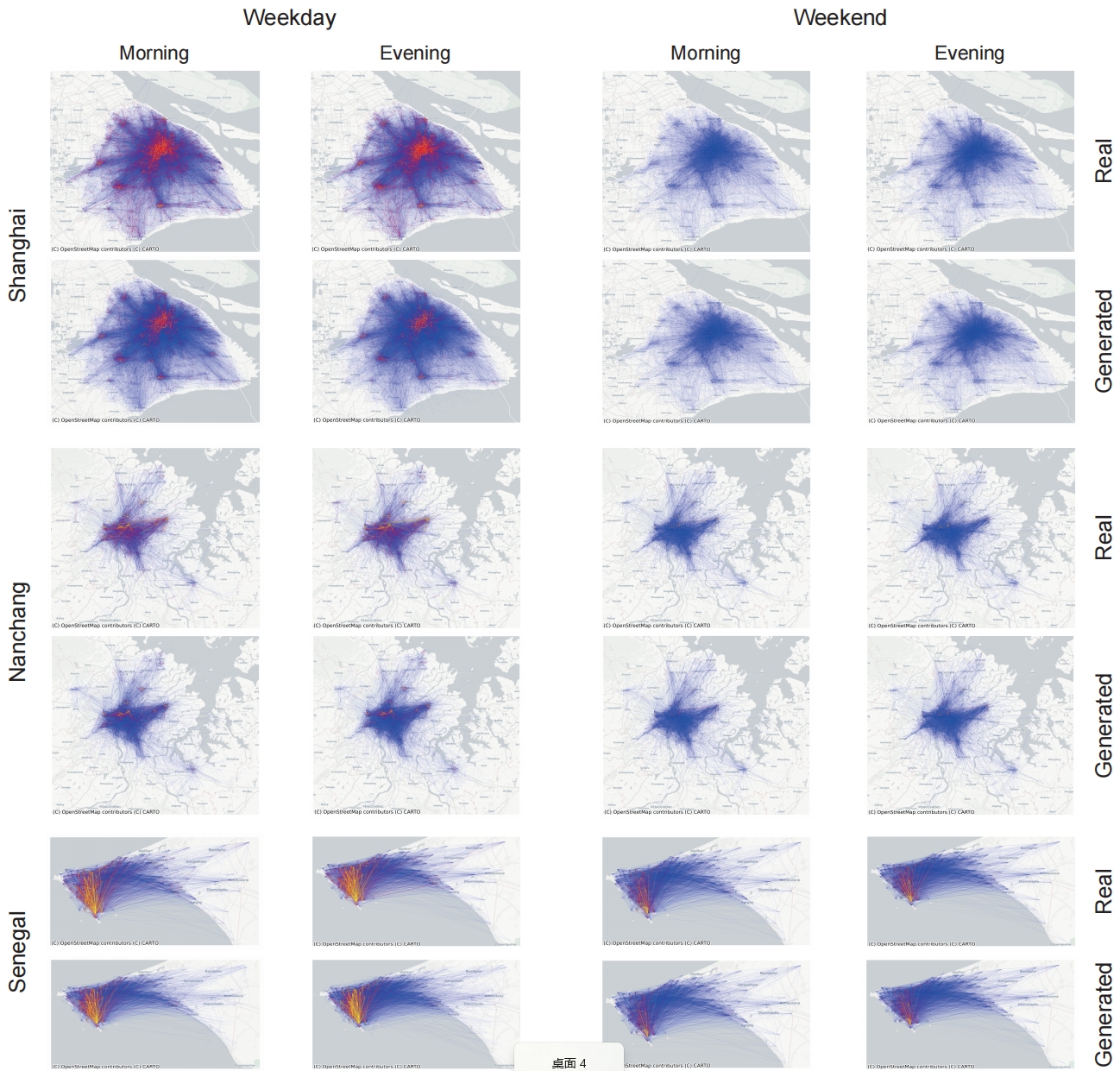
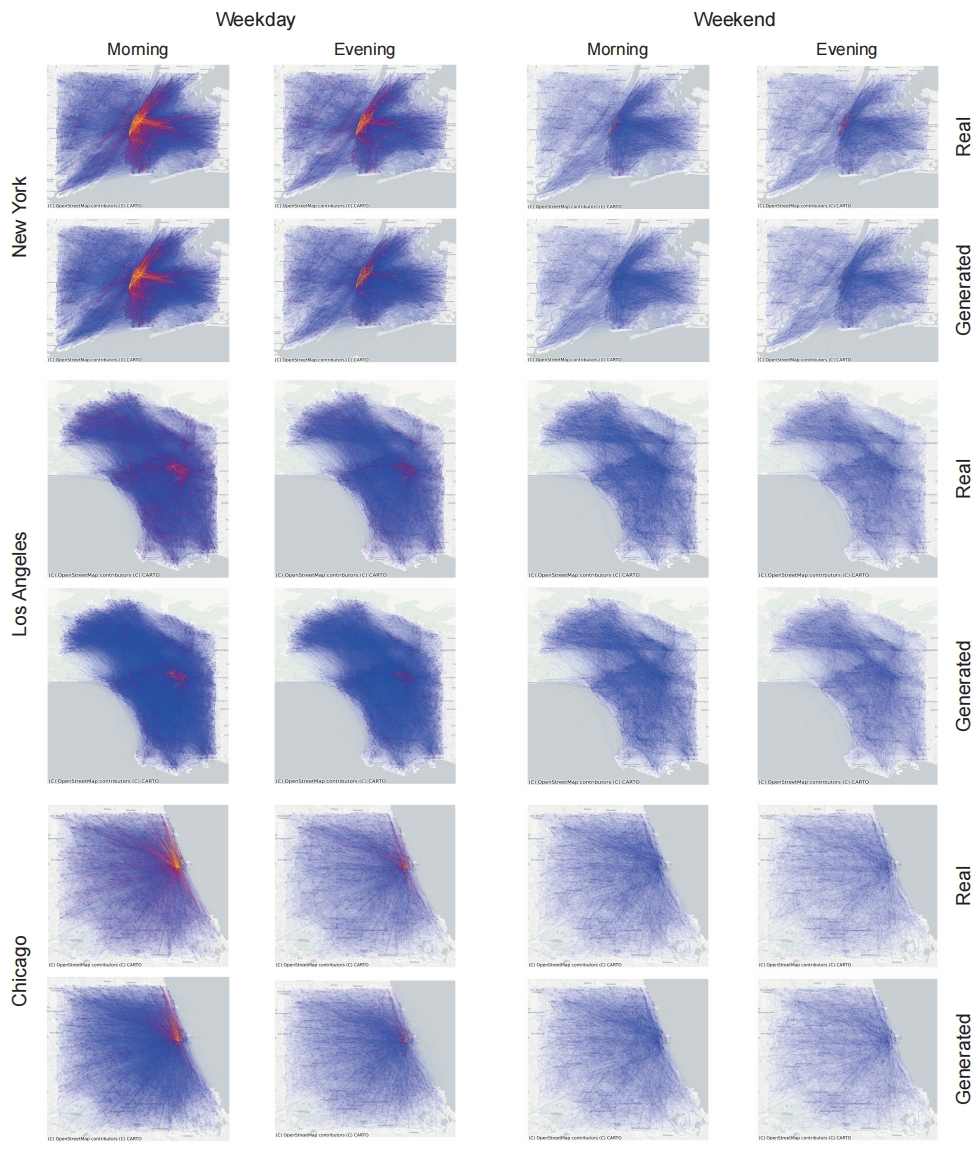
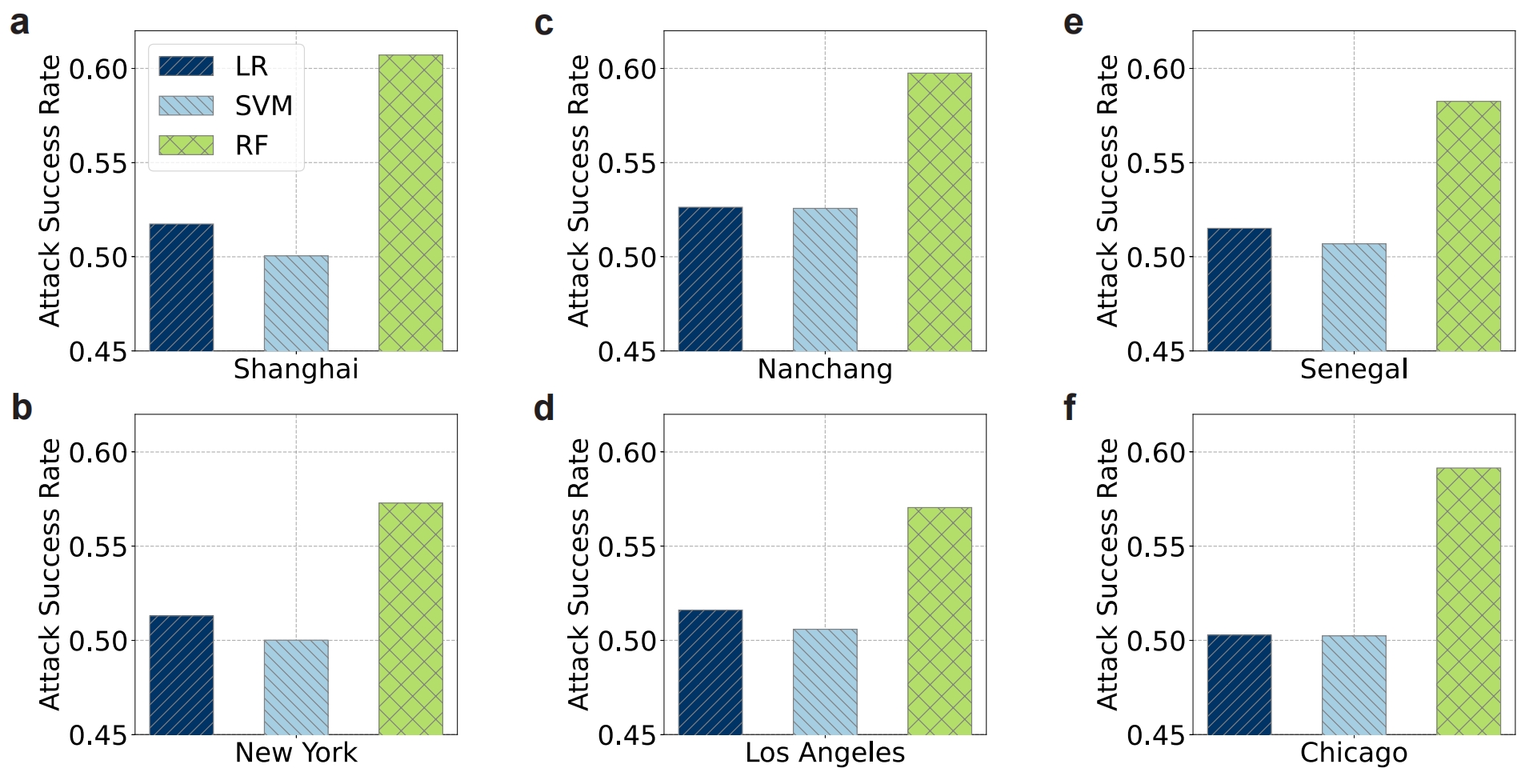
Privacy Protection
As a synthetic global dataset, we evaluate whether the generated data protects individual privacy and avoids potential information leakage. To this end, we conduct a membership inference attack, which is a common method used to assess the risk of privacy breaches. The attack attempts to infer whether a particular data sample was part of the training set, which could indicate potential memorization or leakage of sensitive information. In our evaluation, we train a binary classifier to distinguish between real (training) and synthetic samples based on their latent representations. To ensure the robustness of the results, we adopt three commonly used binary classifiers: logistic regression (LR), support vector machines (SVM), and random forests (RF).